This seminar will take place on November 19 at 15:30, online via Zoom (link below)
https://videoconf-colibri.zoom.us/j/82456512807?pwd=cHRDdldqUWVyTm5RT00ySGgrakl5dz09
Our seminars are free to attend and open to everyone. Please share with whomever may be interested.
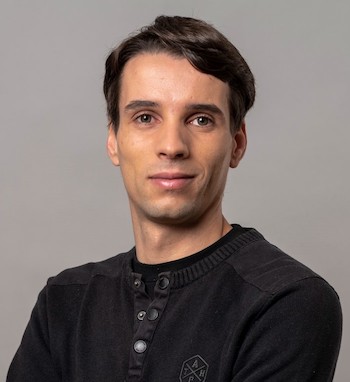
Summary
Seaside operations play a critical role in the world since maritime transportation is the most common way of transporting goods between distant places. Seaside operations are, in practice, highly affected by several uncertainty factors like weather conditions and mechanical failures. Hence, taking uncertainty into account when solution methods are designed is crucial to obtain good quality solutions.
In this talk, we present two of the most important optimization problems under uncertainty arising in seaside operations: the berth allocation and quay crane scheduling problem and the maritime inventory routing problem.
The first problem is studied from a robust optimization point of view, while for the second one several approaches to deal with uncertainty, namely, stochastic programming, robust optimization, risk measure-based models, and safety stock models are considered and compared.
Speaker's bio
PhD in applied mathematics in the field of operations research by the University of Aveiro in 2019, Filipe Rodrigues is currently an assistant professor at Lisbon School of Economics and Management (ISEG), University of Lisbon. He is also a researcher in CEMAPRE – Centro de Matemática Aplicada à Previsão e Decisão Económica. His research mainly focuses on optimization problems (in particular, inventory routing problems) under uncertainty covering different methodological areas such as robust optimization, stochastic programming, risk-measures, and distributionally robust optimization.